
Fairlabs Team
Jan 19, 2024
The ability to analyze large amounts of text data has become increasingly important in today's digital age. From sentiment analysis to natural language processing, there are a wide variety of applications that require algorithms to understand language in a meaningful way. This is where embeddings come in.
Embeddings are a way of turning text into numerical representations that can be analyzed by machines. They make it possible to algorithmically categorize and score text quickly and accurately, allowing us to extract meaning from large volumes of text data.
At its core, an embedding is a mapping between a set of words and a set of vectors. Each word is assigned a unique vector, with the distance between vectors reflecting the similarity between the corresponding words. For example, the vectors for "cat" and "dog" will be more similar to each other than the vectors for "cat" and "table".
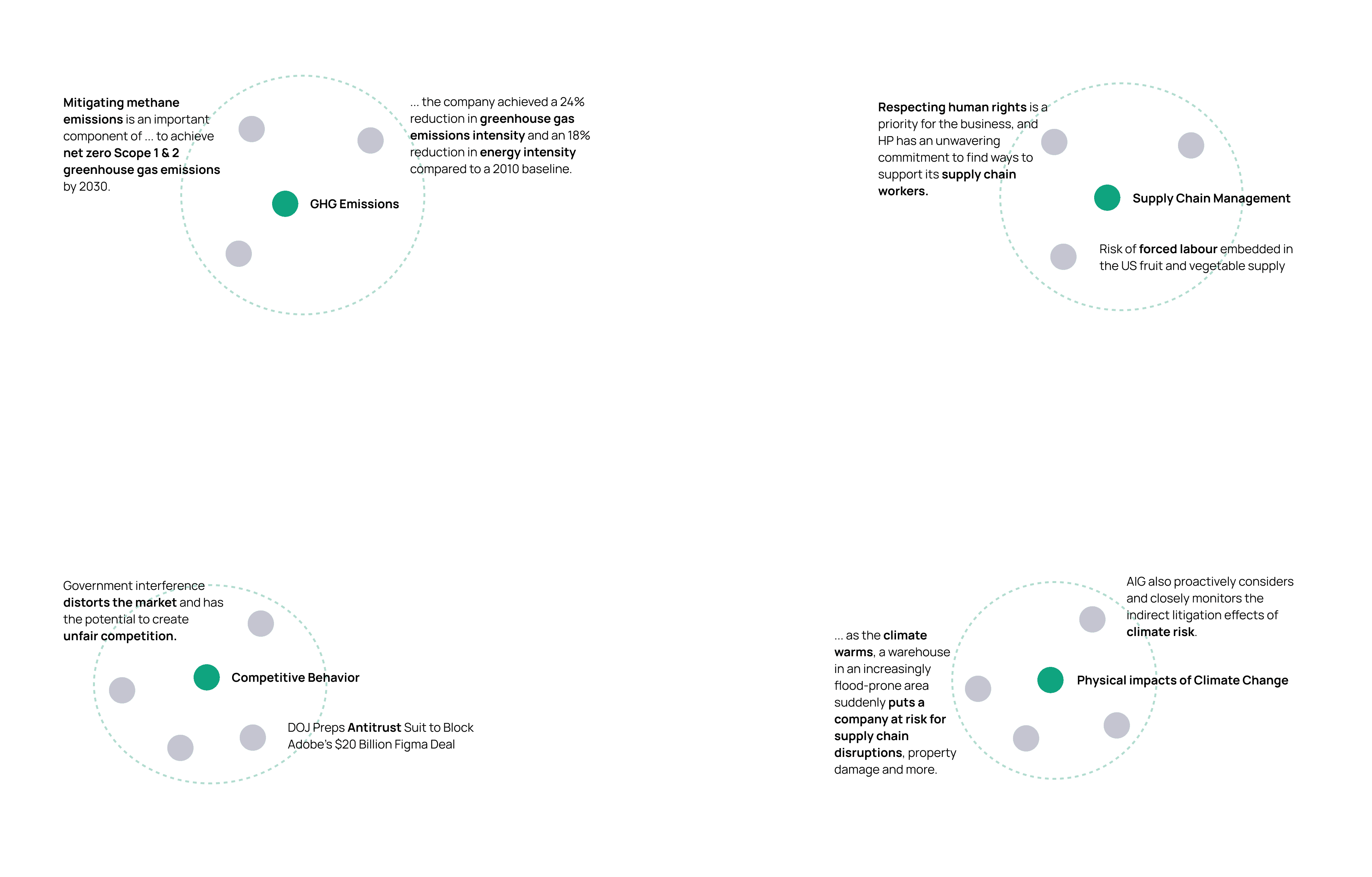
This process of creating embeddings has revolutionized the field of natural language processing. Previously, text data was analyzed using statistical methods, which often required a lot of preprocessing and manual feature engineering. With embeddings, these manual processes can be greatly reduced or even eliminated altogether, allowing algorithms to quickly and accurately analyze large volumes of text data.
One common application of embeddings is sentiment analysis. This involves analyzing large volumes of text data to determine the overall sentiment or emotion of the text. For example, social media platforms may use sentiment analysis to gauge the overall mood of their users or to track the sentiment of their brand.
Another common application of embeddings is language translation. By creating embeddings for different languages, it becomes possible to algorithmically translate text from one language to another. This is particularly useful for businesses and organizations that operate in multiple countries and need to communicate with customers in different languages.
Researchers have also found embeddings to be useful in other applications, such as text summarization, question answering, and document classification. By transforming text into numerical representations, it becomes possible to apply a wide variety of machine learning algorithms to analyze text data and extract meaningful insights.
While embeddings have many benefits, there are also some challenges associated with their use. For example, creating high-quality embeddings requires large amounts of text data and significant computational resources. Additionally, embeddings are highly dependent on the quality of the text data used to create them. Poor quality text data can lead to inaccurate or misleading embeddings.
Despite these challenges, embeddings have proven to be a valuable tool in the analysis of text data. They allow us to algorithmically categorize and score text quickly and accurately, enabling us to extract meaning from large volumes of text data. As the amount of digital text data continues to grow, embeddings are likely to become even more important in the years to come
In short, embeddings are a powerful tool for analyzing text data. By turning text into numerical representations, we can quickly and accurately analyze large volumes of text data, enabling us to extract meaningful insights. While there are some challenges associated with their use, embeddings have already revolutionized the field of natural language processing and are likely to play an increasingly important role in the analysis of text data in the future. As software engineers, data scientists, and researchers, it's important to understand the potential of embeddings and how they can be applied to solve real-world problems.
Latest Posts